Machine Learning in Business Intelligence: Revolutionizing Data-Driven Decision Making
In today’s competitive business landscape, data is a critical asset for organizations across industries. Businesses generate vast amounts of data daily, and the ability to analyze and extract meaningful insights from that data is essential for driving decision-making and gaining a competitive advantage. Business Intelligence (BI), a set of tools and processes used to transform raw data into actionable insights, has traditionally been used to achieve this goal. However, with the rapid advancement of Machine Learning (ML) technologies, the landscape of Business Intelligence is evolving.
Machine Learning enhances Business Intelligence by enabling more accurate predictions, automating complex data analysis tasks, and providing deeper insights into customer behavior, operational efficiency, and market trends. In this article, we’ll explore the integration of Machine Learning in Business Intelligence, its benefits, and how it is reshaping the future of data-driven decision-making.
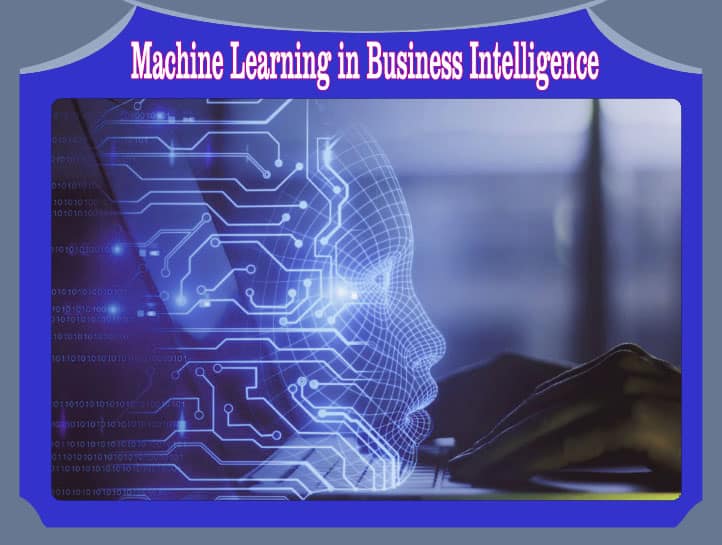
What is Machine Learning?
Machine Learning is a branch of artificial intelligence (AI) that enables systems to learn from data and improve their performance over time without being explicitly programmed. ML algorithms process large datasets, identify patterns, and make predictions or decisions based on the data. Unlike traditional rule-based programming, Machine Learning allows systems to adapt and evolve as they are exposed to more information.
When applied to Business Intelligence, Machine Learning can enhance data analysis by identifying trends, predicting future outcomes, and providing real-time insights that help businesses optimize their strategies.
How Machine Learning Enhances Business Intelligence
The integration of Machine Learning into Business Intelligence unlocks new opportunities for organizations to analyze and leverage their data more effectively. Below are the key ways in which Machine Learning enhances BI processes:
1. Predictive Analytics
One of the most significant contributions of Machine Learning to Business Intelligence is the ability to perform predictive analytics. While traditional BI focuses on historical data to provide insights into past performance, Machine Learning takes this a step further by predicting future trends and outcomes based on past data.
Machine Learning algorithms analyze historical data, identify patterns, and forecast future events, such as customer behavior, sales trends, and market shifts. For example, an e-commerce company can use predictive analytics to forecast customer demand for a specific product based on past purchasing behavior, enabling them to optimize inventory levels and improve sales forecasting accuracy.
2. Automating Data Analysis
In traditional BI systems, data analysis often requires manual input from analysts to clean, process, and interpret the data. This process can be time-consuming and prone to errors. Machine Learning automates many of these tasks, speeding up data processing and reducing the potential for human error.
Machine Learning algorithms can automatically clean and organize data, ensuring that the information being analyzed is accurate and consistent. They can also identify correlations and patterns in the data that would be difficult for human analysts to detect manually. As a result, BI teams can focus on more strategic tasks, such as interpreting insights and making data-driven recommendations.
3. Real-Time Analytics and Decision-Making
Another powerful application of Machine Learning in Business Intelligence is the ability to provide real-time analytics. Traditional BI tools often rely on static, historical data that is updated periodically. While this approach is valuable for long-term trend analysis, it may not be sufficient for businesses that need to make decisions in real time.
Machine Learning algorithms can process data as it is generated, providing organizations with up-to-the-minute insights. For example, a retail company can use real-time analytics to adjust prices dynamically based on real-time market demand, competitor pricing, and customer behavior. This capability allows businesses to make more informed decisions faster, improving their responsiveness and agility in competitive markets.
4. Advanced Customer Insights
Understanding customer behavior is essential for businesses looking to improve customer satisfaction, loyalty, and lifetime value. Machine Learning can analyze large datasets of customer interactions, including purchase histories, browsing habits, and social media engagement, to provide deeper insights into customer preferences and behaviors.
With this information, businesses can segment their customers more accurately, identify key trends, and predict future customer actions. For example, Machine Learning can help marketers identify which customers are most likely to churn or which products are likely to appeal to specific customer segments. This level of insight allows businesses to deliver personalized experiences and marketing campaigns, improving customer engagement and retention.
5. Improved Decision-Making with Prescriptive Analytics
While predictive analytics helps businesses forecast future trends, prescriptive analytics takes it a step further by recommending specific actions based on the data. Machine Learning-powered prescriptive analytics allows BI systems to analyze the potential outcomes of various business decisions and suggest the best course of action.
For example, a supply chain manager can use prescriptive analytics to optimize inventory levels by analyzing factors such as supplier performance, lead times, and demand variability. The system can recommend when to reorder stock, how much to order, and which suppliers to use based on predicted demand and risk factors. This helps businesses optimize their operations and reduce costs.
6. Anomaly Detection and Fraud Prevention
Machine Learning is also playing a crucial role in improving anomaly detection and fraud prevention in Business Intelligence. ML algorithms can continuously monitor large datasets for unusual patterns or behaviors that may indicate fraud, security breaches, or other operational risks.
For instance, financial institutions use Machine Learning to analyze transaction data and detect potentially fraudulent activities in real time. By identifying anomalies such as unusual spending patterns or unauthorized access attempts, businesses can take immediate action to prevent financial losses and protect sensitive information.
Benefits of Integrating Machine Learning with Business Intelligence
The integration of Machine Learning into Business Intelligence systems offers several key benefits for organizations:
1. Enhanced Accuracy and Efficiency
Machine Learning algorithms are capable of processing vast amounts of data quickly and accurately. This leads to more reliable predictions, faster insights, and fewer errors in data analysis. The automation of repetitive tasks, such as data cleaning and pattern recognition, also frees up valuable time for BI professionals, allowing them to focus on high-level strategic analysis.
2. More Informed Decision-Making
By leveraging predictive and prescriptive analytics, Machine Learning allows businesses to make more informed decisions. Whether it’s forecasting future demand, optimizing pricing strategies, or identifying operational inefficiencies, ML-powered BI systems provide data-driven insights that lead to better business outcomes.
3. Personalized Customer Experiences
One of the most impactful benefits of Machine Learning in BI is the ability to deliver personalized experiences to customers. By analyzing customer data in real time, businesses can tailor their offerings, promotions, and communications to individual preferences. This improves customer satisfaction, increases loyalty, and drives higher sales.
4. Scalability
As businesses grow and generate more data, the scalability of their BI systems becomes a critical concern. Machine Learning enables organizations to scale their data analysis efforts efficiently. Whether analyzing small datasets or processing petabytes of information, ML algorithms can handle the complexity and size of modern data environments, making them ideal for organizations of all sizes.
5. Proactive Risk Management
Machine Learning helps organizations identify potential risks before they become significant problems. By continuously analyzing data for anomalies and unusual patterns, ML algorithms can alert businesses to potential risks in real time, allowing them to take proactive measures to mitigate these risks. This is particularly valuable in areas such as cybersecurity, fraud detection, and operational efficiency.
Challenges and Considerations for Machine Learning in Business Intelligence
While the benefits of Machine Learning in Business Intelligence are clear, there are also challenges and considerations that organizations must address:
1. Data Quality
The accuracy of Machine Learning models depends heavily on the quality of the data being analyzed. Poor-quality data, such as incomplete, inconsistent, or inaccurate information, can lead to incorrect predictions and insights. Organizations need to invest in robust data governance and data management practices to ensure that their data is clean and reliable.
2. Data Privacy and Security
As Machine Learning systems process large amounts of sensitive data, organizations must ensure that they comply with data privacy regulations, such as the General Data Protection Regulation (GDPR). Additionally, they must implement strong security measures to protect their data from unauthorized access and breaches.
3. Skill Gaps
Implementing Machine Learning in Business Intelligence requires specialized skills in data science, machine learning algorithms, and data engineering. Many organizations may face challenges in finding and retaining the right talent to develop, implement, and maintain ML-powered BI systems.
4. Complexity
Machine Learning models can be complex and difficult to interpret, especially for non-technical stakeholders. BI teams need to focus on making the insights generated by ML systems accessible and understandable to decision-makers across the organization.
The Future of Machine Learning in Business Intelligence
The future of Machine Learning in Business Intelligence is bright. As ML technologies continue to evolve, we can expect even more sophisticated applications in areas such as natural language processing (NLP), advanced predictive analytics, and automated decision-making.
Moreover, the increasing availability of cloud-based AI and ML platforms is making these technologies more accessible to businesses of all sizes. Organizations that invest in integrating Machine Learning into their BI systems will be well-positioned to remain competitive in an increasingly data-driven world.
Conclusion
Machine Learning is revolutionizing Business Intelligence by enhancing data analysis, enabling predictive and prescriptive analytics, and automating complex tasks. The integration of ML in BI empowers businesses to make faster, more accurate decisions, personalize customer experiences, and optimize operations in real time. While challenges such as data quality and skill gaps remain, the potential of Machine Learning to transform Business Intelligence is undeniable. By embracing ML-powered BI systems, organizations can unlock new opportunities for growth, innovation, and success in the digital age.